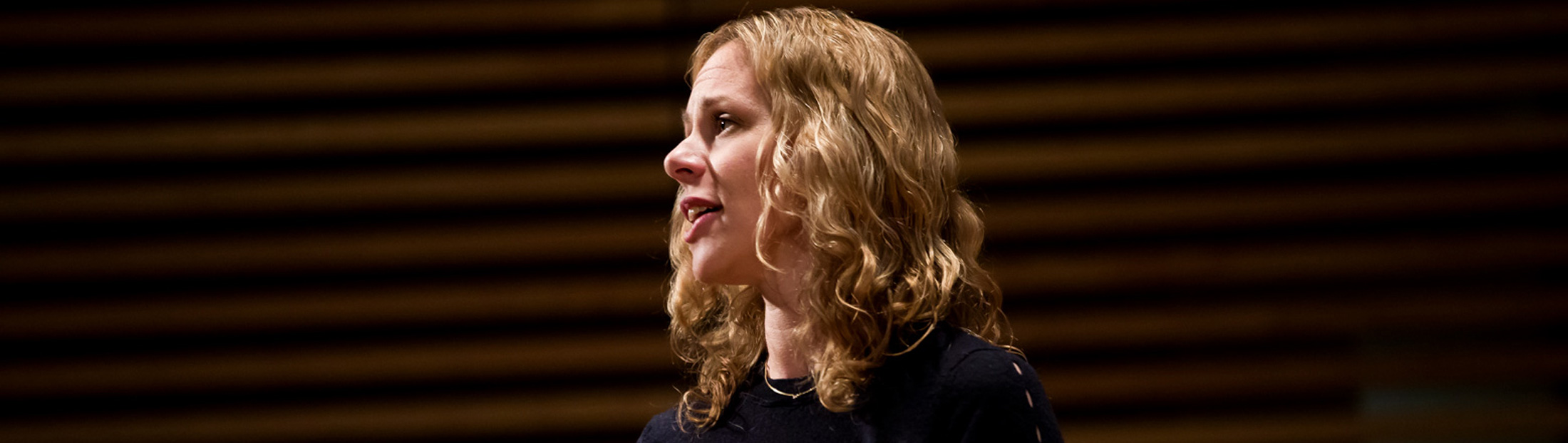
Detecting Huntington’s disease with an algorithm that analyzes speech
New, preliminary research found automated speech test accurately diagnoses Huntington’s disease 81 percent of the time and tracks the disease’s progression.
New, preliminary research found automated speech test accurately diagnoses Huntington’s disease 81 percent of the time and tracks the disease’s progression.
In an advance that could one day provide new insight into the progression of neurological diseases like Huntington’s disease, Alzheimer’s and Parkinson’s, researchers have demonstrated the first automated system that uses speech analysis to detect Huntington’s disease.
A team of University of Michigan and Michigan Medicine researchers demonstrated that the system, which analyzes a recording of a pre-selected passage, can effectively detect Huntington’s disease 81 percent of the time, as well as track its progression.
The work could power future systems that monitor patients continuously, providing doctors and researchers with far more detailed information than is available today. There currently is no cure for Huntington’s disease, which affects approximately 30,000 Americans, with 200,000 more facing a genetic risk of developing the disease.
While speech analysis is already used to track the disease’s progression, it’s an expensive and time-consuming process that requires patients to visit a clinic and record speech for manual analysis. Emily Mower Provost, a U-M computer science and engineering associate professor who leads the work, says an automated system would could improve patient care and our understanding for how disease symptoms change over time.
“We see our technology providing a mechanism to augment care. In-clinic assessments provide only brief snapshots of symptoms,” she said. “An automated system could give doctors a much more fine-grained analysis of symptom changes. It could also help patients gain more insight into their own condition.”
Ultimately, she envisions a smartphone-based system that could continuously record patients’ speech and analyze it in real time to provide an ongoing picture of disease progression that could be accessed by both patients and doctors. The data could also be combined into a rich trove of anonymized information that could help drug makers and other researchers develop a better fundamental understanding of the disease.
Automated speech recognition systems are common today, but Mower Provost explains that off-the-shelf systems can’t be used for patients with Huntington’s disease because their systems are trained on healthy speech. The telltale speech symptoms of Huntington’s disease—like stuttering, mispronouncing and pausing for long periods—introduce errors that make the systems perform poorly.
An automated system could give doctors a much more fine-grained analysis of symptom changes. It could also help patients gain more insight into their own condition.”
Emily Mower Provost, Assistant Professor of Electrical Engineering and Computer Science
So, the team partnered with clinicians at Michigan Medicine to create their own system designed to transcribe the unique speech patterns of patients with Huntington’s disease. They then used the system’s output to create a set of measures that can predict the disease.
“Designing algorithms is only one aspect of this kind of work—if you want to innovate on the algorithmic side, you need to understand the clinical domain,” she said. “If you don’t do that, your algorithms are going to have limited clinical effectiveness.”
While Mower Provost cautions that the work in the paper is preliminary, she says it’s an important first step that provides a path to designing automated speech analysis systems that can identify and track neurological disease.
The next step is to refine the system to make it work outside the controlled conditions of the lab, enabling it to recognize disease indicators in spontaneous speech in a variety of settings. Because dysarthria and apraxia are symptoms of other neurological diseases as well, Mower Provost believes that the research may help build better understanding of a variety of diseases in the future.
The researchers present a paper on the work at the Interspeech conference in Hyderabad, India on Sept. 5. The paper is titled “Classification of Huntington’s Disease using Acoustic and Lexical Features.” The research was supported by the National Institutes of Health’s National Center for Advancing Translational Sciences and by the National Science Foundation.