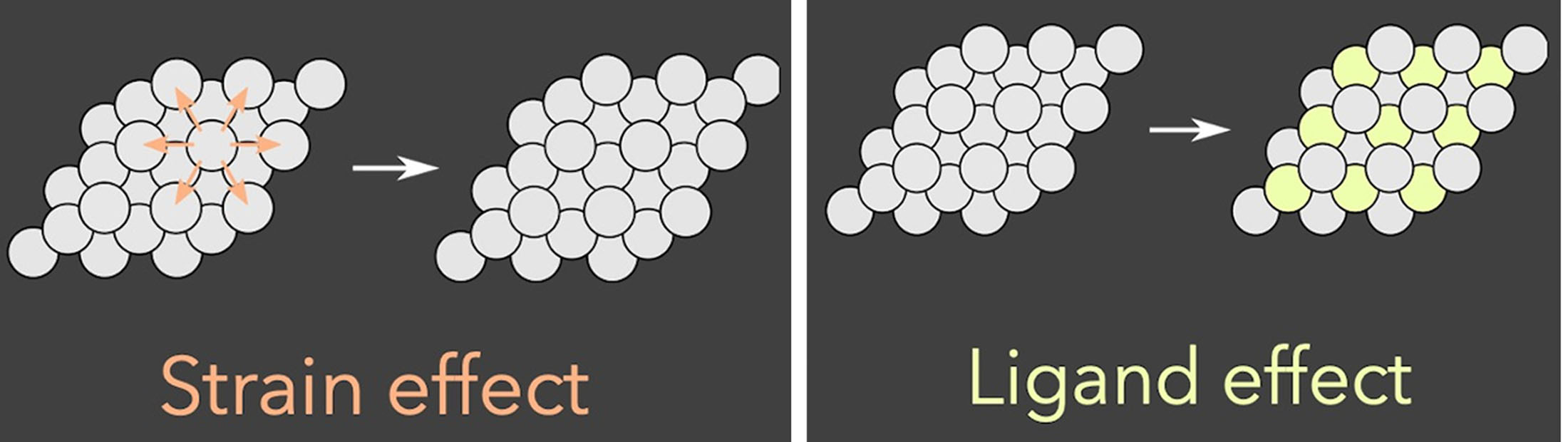
Chemistry and energy: Machine learning to understand catalyst interactions
Toward harnessing machine learning to design the materials we want.
Toward harnessing machine learning to design the materials we want.
A machine learning technique rapidly rediscovered rules governing catalysts that took humans years of difficult calculations to reveal—and even explained a deviation. The team, based at the University of Michigan, believes that other researchers will be able to use the same technique to make faster progress in designing materials for a variety of purposes.
“This opens a new door, not just in understanding catalysis, but also potentially for extracting knowledge about superconductors, enzymes, thermoelectrics, and photovoltaics,” said Bryan Goldsmith, an assistant professor of chemical engineering, who co-led the work with Suljo Linic, a professor of chemical engineering.
The key to all of these materials is how their electrons behave. Researchers would like to use machine learning techniques to develop recipes for the material properties that they want. For superconductors, the electrons must move without resistance through the material. Enzymes and catalysts need to broker exchanges of electrons, enabling new medicines or cutting chemical waste, for instance. Thermoelectrics and photovoltaics absorb light and generate energetic electrons, thereby generating electricity.
Machine learning algorithms are typically “black boxes,” meaning that they take in data and spit out a mathematical function that makes predictions based on that data.
“Many of these models are so complicated that it’s very difficult to extract insights from them,” said Jacques Esterhuizen, a PhD student in chemical engineering and first author of the paper in the journal Chem. “That’s a problem because we’re not only interested in predicting material properties, we also want to understand how the atomic structure and composition map to the material properties.”
But a new breed of machine learning algorithm lets researchers see the connections that the algorithm is making, identifying which variables are most important and why. This is critical information for researchers trying to use machine learning to improve material designs, including for catalysts.
A good catalyst is like a chemical matchmaker. It needs to be able to grab onto the reactants, or the atoms and molecules that we want to react, so that they meet. Yet, it must do so loosely enough that the reactants would rather bind with one another than stick with the catalyst.
In this particular case, they looked at metal catalysts that have a layer of a different metal just below the surface, known as a subsurface alloy. That subsurface layer changes how the atoms in the top layer are spaced and how available the electrons are for bonding. By tweaking the spacing, and hence the electron availability, chemical engineers can strengthen or weaken the binding between the catalyst and the reactants.
Esterhuizen started by running quantum mechanical simulations at the National Energy Research Scientific Computing Center. These formed the data set, showing how common subsurface alloy catalysts, including metals such as gold, iridium and platinum, bond with common reactants such as oxygen, hydroxide and chlorine.
The team used the algorithm to look at eight material properties and conditions that might be important to the binding strength of these reactants. It turned out that three mattered most. The first was whether the atoms on the catalyst surface were pulled apart from one another or compressed together by the different metal beneath. The second was how many electrons were in the electron orbital responsible for bonding, the d-orbital in this case. And the third was the size of that d-electron cloud.
The resulting predictions for how different alloys bind with different reactants mostly reflected the “d-band” model, which was developed over many years of quantum mechanical calculations and theoretical analysis. However, they also explained a deviation from that model due to strong repulsive interactions, which occurs when electron-rich reactants bind on metals with mostly-filled electron orbitals.
The paper is titled, “Theory-guided machine learning finds geometric structure-property relationships for chemisorption on alloys.”
The work was supported by the Department of Energy.